The Future of AI: More Human Than We Think?
By Rob Boerman | Published on 2024-09-23
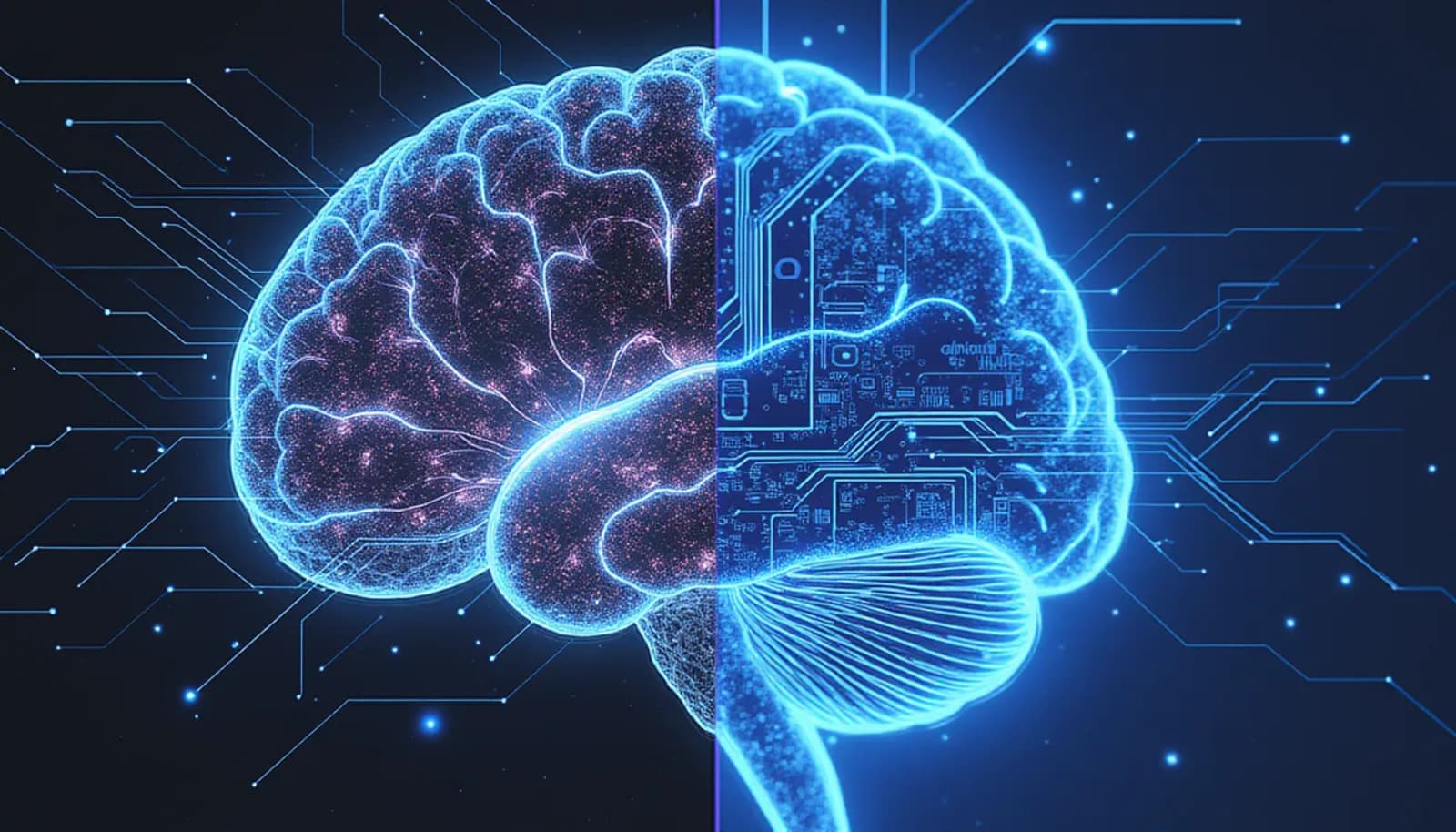
Over the past 2 years we have been bombarded with a million cool new AI tools and language models. Yet at the same time enterprises are struggling making it work for them. Implementations usually fail on bad quality output or on concerns about the reliability and explainability of the systems reasoning. But is that so strange when the core architecture was inspired by the human brain and reasoning?
Ever since I learned about neural networks and AI, I have been fascinated by how a simplified implementation of the brain's architecture could lead to such powerful computational systems. What captivates me even more is the realization that there's so much more to learn from our minds than just their physical structure. The intricate ways our brains process information, make decisions, and solve problems offer a wealth of inspiration for advancing AI.
As I delved a bit deeper, I noticed that the parallels between artificial and biological intelligence extend far beyond mere structural similarities. Psychologist Kahneman describes a framework of System 1 (fast, intuitive) and System 2 (slow, deliberate) thinking. This framework provides an interesting lens through which we can examine parallels with AI systems, especially when compared with recent AI advancements like OpenAI's GPT-o1 model.
Let's explore how these two systems of thinking manifest in both human cognition and AI:
System 1 Thinking: Our Cognitive Autopilot
Imagine you're catching up with an old friend over coffee. As you chat, you effortlessly understand their words, pick up on their tone, and respond with your own thoughts and feelings. This smooth, almost automatic interaction is System 1 thinking in action.
It's our cognitive autopilot, which allows us to swiftly process the world around us. System 1 is the reason we can instantly recognize a friend's face in a crowd or instinctively pull our hand away from a hot stove. It's quick, intuitive, and draws upon a vast reservoir of experiences and learned patterns.
Like our System 1, LLMs are trained on enormous amounts of data, being able to swiftly identify patterns and generate responses based on their training. The context-sensitivity of System 1 thinking is also a bit similar to how LLMs generate responses based on the immediate context of the prompt. Just as our gut reactions can be influenced by emotions and prone to biases, LLMs can reflect biases present in their training data or come up with responses that lack deeper reasoning.
System 2 Thinking: Our Inner Problem Solver
Now, imagine you're a project manager planning a major product launch. As you tackle this complex task, you engage in deep, deliberate thought – this is System 2 thinking in action. You're not relying on intuition, but are methodically analyzing each aspect: market research, timelines, budgets, and strategies.
This analytical thinking is the hallmark of System 2. It's slower and requires more effort than our intuitive System 1, but it allows us to handle abstract concepts and complex challenges with precision. System 2 enables us to think flexibly, question assumptions, and imagine new possibilities.
Fascinatingly, we're seeing AI systems attempt to mirror this kind of deliberate thinking. The recent unveiling of OpenAI's o1 model is a prime example of this shift towards System 2-like thinking in AI. The o1 model is designed to "spend more time thinking before it responds," allowing it to tackle more challenging problems in fields like science, coding, and math.
Some of the features of OpenAI GPT-o1 that mimic System 2 thinking include:
- Chain-of-thought reasoning: The model goes through multiple steps of reasoning, and deconstructs complex problems into manageable parts, before providing an answer, similar to how we usually work through a problem methodically.
- Reduced hallucinations: By taking more time to process and reason, o1 is less likely to generate false or irrelevant information, much like how our deliberate thinking helps us avoid jumping to conclusions.
- Improved performance on complex tasks: o1 excels at tasks requiring detailed reasoning, which aligns with the strengths of System 2 thinking.
This approach does mean slower response times compared to previous models, but the trade-off is potentially more accurate and well-reasoned outputs. This mirrors how System 2 thinking in humans is slower but often more reliable for complex problem-solving than our quick, intuitive System 1 responses.
An Exciting Journey Ahead
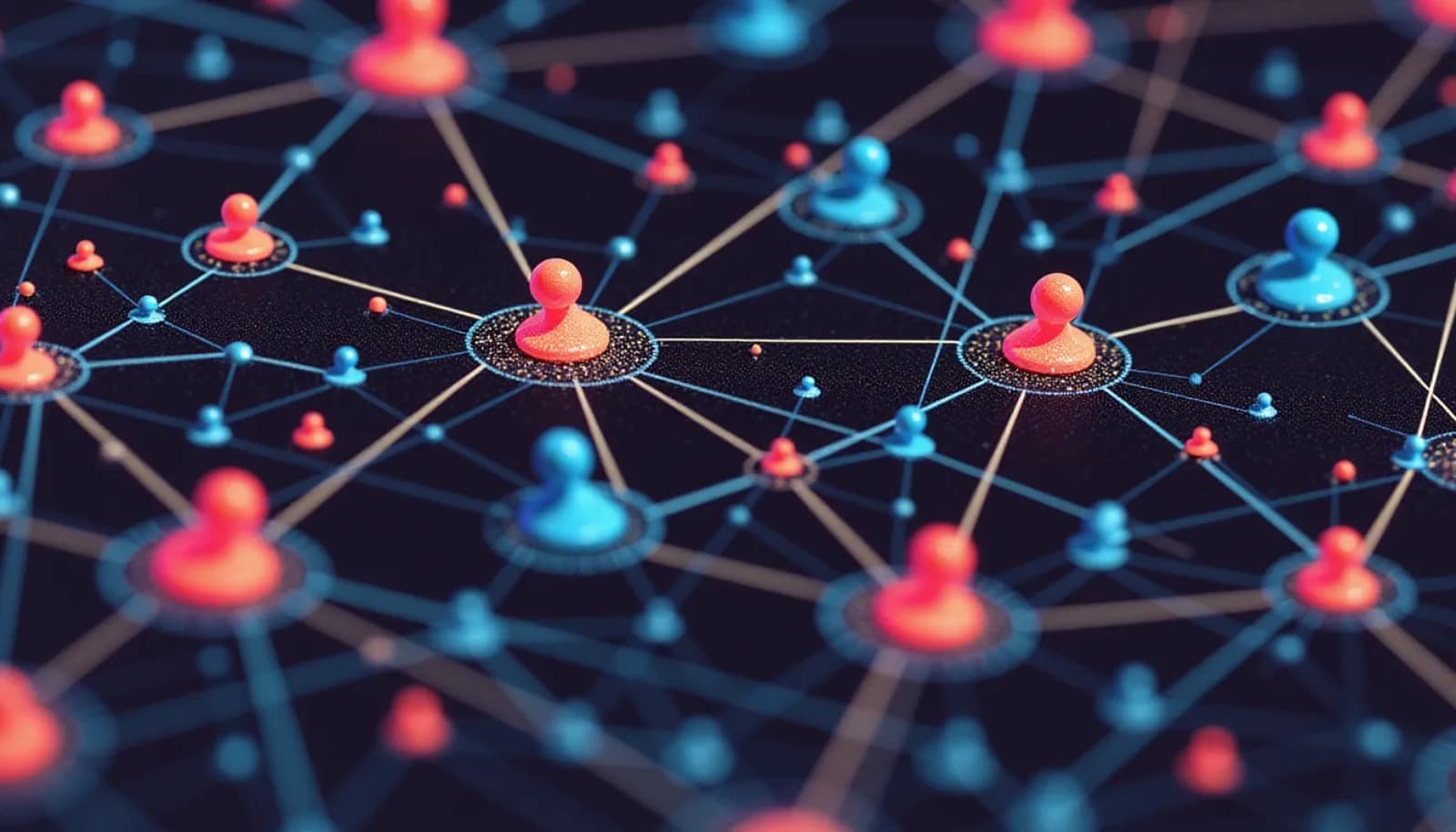
As AI continues to evolve, we're seeing attempts to combine the strengths of both System 1 and System 2 approaches. Advanced AI systems are being developed that can switch between fast, intuitive responses and slower, more deliberate reasoning, much like how we integrate these two modes of thinking in our everyday lives. The o1 model is a great example of this trend, showing how AI can benefit from not trying to solve everything with a perfectly trained model, but instead focusing more on reasoning and self-correction abilities.
But what if we took inspiration not just from individual thinking, but from how humans work together? While the development of more sophisticated language models is undoubtedly valuable, I've found that some of the most promising advancements come from a different approach. Instead of solely focusing on creating all-encompassing models, we're seeing remarkable results by exploring how different AI agents—each with unique personas, tools, and training—can interact and collaborate.
This agentic approach to AI mirrors human collaboration in fascinating ways. Just as teams of specialists often outperform individual generalists in complex tasks, we're finding that systems of specialized AI agents can achieve outcomes that surpass the capabilities of even the most advanced single models, especially when humans are involved in the critical steps.
The journey ahead is exciting, and I look forward to sharing more insights as we continue to push the boundaries of what's possible in AI. The future of enterprise AI isn't just about bigger models—it's about smarter, more collaborative systems that can truly transform the way we work and innovate.